When someone asks, “Do you get my drift?” the intent is to make sure the other person understands.
But when “drift” comes up in the context of artificial intelligence, the meaning is a lot closer to an evolution away from common understanding.
AI needs to be trained before it can begin doing its job. But as with many forms of education, the information can become outdated, sometimes very quickly.
“Drift” is the term AI practitioners use to describe instances where the way the technology functions or the vocabulary used for its training is no longer current, causing a gulf between what the software has been trained to do and what institutions expect it to do.
Several AI practitioners in financial services shared their insights on challenges like this during a panel discussion about the technology. They also talked about tackling AI bias, using digital twins and dealing with high margins of error.
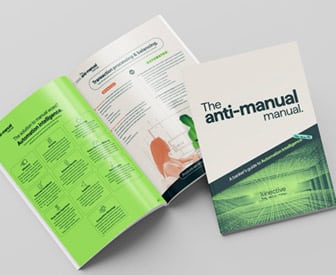
The New AI: A Banker’s Guide to Automation Intelligence
Manual tasks across channels is costly. And while AI is hot, there’s a simpler way to bring efficiency that many bankers have overlooked.
Read More about The New AI: A Banker’s Guide to Automation Intelligence
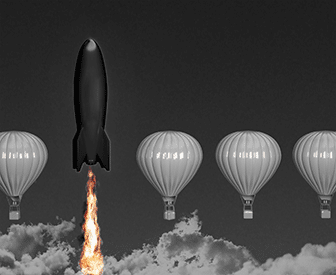
The unfair advantage for financial brands.
Offering aggressive financial marketing strategies custom-built for leaders looking to redefine industry norms and establish market dominance.
How AI Drift Impacts Results and Creates Risks
The Federal Reserve Bank of New York is among those that have run into challenges with the specialized language models underlying its AI systems.
“As you can imagine, the financial language that’s being used changes over time,” said Harry Mendell, data architect specializing in artificial intelligence at the New York Fed. The terms “omnichannel” and “embedded banking,” for example, don’t mean exactly what they did five years ago, and even now, humans don’t always agree on what they mean.
“We had to keep retraining our models,” said Mendell.
It became clear that continuous training made more sense, so the New York Fed now strives for weekly updating.
Want to go deep on AI best practices for banks?
Attend our AI Masterclass — Unlocking the Power of Artificial Intelligence in Banking — at The Financial Brand Forum 2024 on May 20-22 in Las Vegas. Led by Ron Shevlin, chief research officer at Cornerstone, this three-hour workshop will be jam-packed with lessons learned from industry leaders and real-world case studies.
For more information and to register, check out the Forum website.
Suresh Ande, director of global markets risk analytics at Bank of America Merrill Lynch, and Ercan Ucak, a vice president at Cerberus Capital Management, agreed that frequent retraining of artificial intelligence technology is necessary. All three executives participated in an event that Re•Work hosted in the spring to allow AI practitioners in financial services to share their experiences — and lessons learned — with each other.
The structure of AI models can reflect the way a particular process was first designed, Ucak said. Sometimes processes can change sufficiently enough that retraining in the basics may be necessary.
Another aspect of drift — which calls to mind HAL from the 1968 film “2001: A Space Odyssey” — happens inside the technology. Sophisticated AI models can migrate forward from their original programming, according to Techopedia. One notable result, the website recounted, was when two Facebook chatbots began to “talk” to each other several years ago in a secret code not envisioned by their builders.
The idea of drift comes up in other contexts in financial services as well, such as concerns that, over time, AI used for credit evaluation can pick up biases that can lead to discrimination.
Read more: Ally Taking ChatGPT Slow, But Could Be Using It By Yearend
How Do You Measure Return on Investment from Artificial Intelligence?
The banking industry has seen a virtual explosion of use cases for artificial intelligence, a trend hastened by the belief in its potential to boost revenue, increase production, improve efficiency, and otherwise generate benefits that go far beyond what can be achieved with traditional technology.
However, the three panelists at the AI in Finance Summit — which focused on exploring the challenges of adopting this technology in financial services — made it clear that there are detours between the adoption of AI and the eventual benefits.
Even determining the return on investment for an AI implementation is not a straightforward matter. Cerberus Capital’s Ucak told listeners that establishing key performance indicators up front is essential to understanding what effect an AI application might be having. To make that happen, a governance structure has to be put in place to ensure consistent measurement and evaluation.
Ande of BofA Merrill Lynch said that some types of ROI can be more easily measured than others. For example, let’s say a bank decides to use AI-based computer code generation tools to speed up programming time. If the process usually takes 10 days and AI cuts it to five days, a clear increase in productivity has resulted, he said.
Mendell said that the New York Fed’s supervisory division has used AI to assist with bank examinations. “We were able to have examiners complete their work in a matter of days instead of weeks,” he said. That’s a clear gain in productivity.
At a traditional commercial bank, AI might deliver similar benefits when used for an internal loan review or a compliance audit.
But assessing the return on investment is trickier in cases where artificial intelligence is used for customer interactions, Ande noted. A lot would depend on the metric being used.
For example, both retention and satisfaction affect the bottom line. Measuring customer retention is straightforward — people stay or they leave. But assessing customer satisfaction would require qualitative research to see how happy or unhappy people were.
In addition, if an AI-driven process alienates some customers and they complain on social media, how should that reputational erosion be factored into ROI calculations?
“It’s very difficult,” Ande said, “to measure things in terms of negativity.”
Read more:
- A Banker’s Guide to Digital and AI Transformation Success
- Pros & Cons of ChatGPT and Other ‘Generative AI’ for Marketers

What Are ‘Digital Twins’ in the Banking Space?
One of the many interesting topics that came up in the panel discussion is the concept of digital twins.
A digital replica of retired basketball star Carmelo Anthony is a fun application of the concept, said Ande.
Soul Machines, a company that designs avatars of people for use in the metaverse, created the AI version of Anthony. It makes “Digital Melo” available for influencer appearances on social media and even for appearances with the real Anthony himself.
Business-oriented digital twinning is not as fun in spirit yet is meaningful in results.
“You basically look at your business and then develop a digital counterpart of it — a clone,” said Ucak. These twins can range from very elaborate to cursory. At the far end, internet-of-things devices and other monitors can be tied in for added realism. “You can go all out, having a lot of devices everywhere,” said Ucak.
Mendell said the New York Fed has been using a digital twin approach to better manage its currency distribution function. Using AI technology for that has made it more efficient.
Read more:
- Failing to Experiment with AI Tools Like ChatGPT Is a Big Mistake
- The Hidden Risks of Artificial Intelligence in Bank Marketing

Can Banking Tolerate ‘Nearly Right’ Results from AI?
The horrifying ChatGPT gaffes that have surfaced in the media make an easy target for those wary of artificial intelligence’s growing societal influence. But major mistakes are not the only red flag.
Software that doesn’t get things quite right is a big problem for financial institutions.
“The financial industry is based on accuracy and trust. We are so used to that. Now suddenly AI comes along and gives 85% accuracy. That doesn’t help much.”
— Suresh Ande, Bank of American Merrill Lynch
As Ande puts it, the mindset for most aspects of financial services is “binary” — the results are right or they are wrong. Typically, if there’s a 15% margin for error, there will be risk management issues and likely reputational damage, he said.
Mendell said that his experience working with AI has been that top management is more tolerant of human error than technology error.
“It’s far more acceptable psychologically for people to make judgment errors than having a machine make a judgment error,” he said.
“It’s hard being held to a higher standard than a human, but it happens.”
— Harry Mendell, New York Fed
Something that can clearly cause sleepless nights is the “black box” of deep learning, wherein AI “ponders” its “experiences” and adjusts accordingly.
Ande said that with statistical models that run processes, there is some visibility. But “when you enter into deep learning, explainability becomes opaque,” he said. “And that’s a big challenge for financial services. It’s kind of cryptic.”
See all of our latest coverage of artificial intelligence in banking.
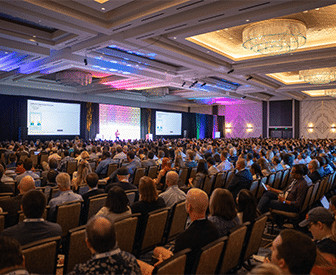
Join industry’s leading AI conference - free passes available!
Ai4 is coming to Las Vegas, August 12-14 2024. Join thousands of executives and technology innovators at the epicenter of the AI community.
Read More about Join industry’s leading AI conference - free passes available!

Why Industry Cloud for Banking?
PwC’s Industry Cloud for Banking helps deliver personalized products and services that today’s customers expect.
Synthetic Data: A Way to Address Concerns About AI Bias?
The possibility of bias developing when artificial intelligence is used in lending — some warn it is virtually certain over enough time — remains a continuing concern. One errant loan officer can only hurt so many applicants before something triggers an inquiry. But AI technology is used to process loans en masse and can affect many borrowers in a short time.
Ande said that synthetic data can help. This is information that’s created using algorithms. TechTarget says it is “used as a stand-in for test data sets of production or operational data, to validate mathematical models and to train machine learning models.”
“You can work with synthetic data and use it to try to create some kind of mitigator,” said Ande. Using synthetic data can also address privacy concerns when using real data to train or test AI technology could result in unauthorized developers seeing customers’ private information.