Many banking institutions have invested in artificial intelligence for better insights and improved business decisions. AI has primarily been used as an extension of traditional analytics to work through thousands of data points and to determine which customers are most likely to respond to a particular offer or to surface patterns and trends within those segments. In this way AI’s most common use has been to drive cross-selling and to identify customers who may leave.
But AI has significantly more potential. Banks are finding that just because a set of customers are in the same segment does not mean they act the same way or have similar preferences. In reality, there will be multiple actions relevant to each individual.
Today’s customers demand a seamless and personalized experience in every channel and interaction. They expect to have a tailored experience and not to be treated as just a lookalike customer in a pre-determined segment. In addition, it is critical to consider a customer’s actions and reactions to offers across channels to provide a consistent experience and demonstrate recognition of all responses.
Most importantly, offers made to customers must not be solely focused on cross-selling, but based on the possibility that the best action may be to help them resolve a service issue or simply provide information that could help nurture the relationship.
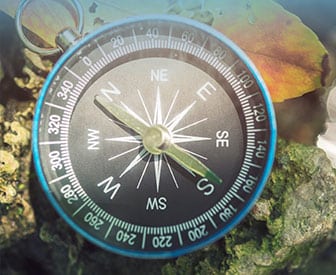
Are You Ready for a Digital Transformation?
Unlock the potential of your financial institution's digital future with Arriba Advisors. Chart a course for growth, value and superior customer experiences.
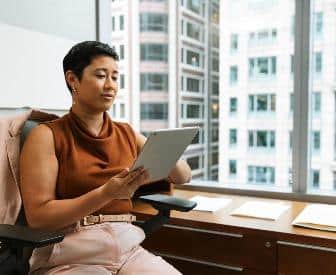
Industry Cloud for Banking from PwC
PwC’s Industry Cloud for Banking applies our deep industry knowledge to your specific business needs
AI Helps Meet Customer Demand for Personalization
Determining which action is most relevant to an individual customer is challenging. The process involves understanding the real-time context and history of the customer across every channel and interaction, and then evaluating the hundreds of potential actions or conversations that customer might be eligible for to determine the best outcome.
This means having a single decision engine or “source of truth” rather than multiple decisionmaking tools embedded in disparate channels and product lines.
AI Covers Range of Interactions:
Being able to deliver a truly personalized experience that customers increasingly demand will depend on an organization's ability to mobilize AI-powered decisionmaking in every channel, in-bound and out-bound, and in real-time.
For example, if a customer’s payment didn’t go through, or they received a late fee that they disagree with, natural language processing can be used to detect feelings of frustration and alert an agent to step in pre-emptively. The agent might offer a fee waiver while the customer is still logged in to online banking or mobile banking. Traditional in-channel decisionmaking would mean waiting for the data to trigger an outbound communication, potentially using a different channel than the one the customer is interacting in.
All of this may be too late to avoid a customer defection.
Wells Fargo leveraged real-time modeling and adaptive machine learning to constantly recalculate the “next best conversation” for each client. This happens while they are interacting in-channel. This not only ensures each customer message is relevant, but the adaptive modeling and AI continue to learn and help the bank get “smarter” with every interaction. Wells Fargo has seen customer engagement rates increase by a factor of three to five, depending on the channel and use case, with conversion rates increasing across channels.
Read More:
- Can Artificial Intelligence Jeopardize Banks’ Loan Growth?
- Why Data and Artificial Intelligence Will Transform the Future of Banking
- Artificial Intelligence Could Make Bank Overdraft Fees Obsolete
Overcoming Organizational Challenges Through AI
Most banks continue to operate in cultural and operational silos, with marketing and commercial teams often competing to get their offerings in front of customers. This has encouraged investment in disparate point solutions designed to optimize single channels or product lines, leaving others disconnected.
The latest research shows that today’s customers are much less tolerant of disjointed and inconsistent experiences and more likely to defect to those institutions better able to meet their personal needs.
Centralized decisionmaking is an effective way to overcome these operational silos and unite technology stacks without having to re-build core and costly infrastructure. This can be used to unite thousands of data points that may reside on different systems and arbitrate the best outcome, channel and treatment at that moment, without the need for human input. When applied appropriately, it can leverage existing analytic models and provide feedback loops for continuous improvement.
AI Focuses Bank Efforts:
Using self-learning models means recommendations become more accurate and relevant with every interaction. Human error and the costly overhead of continuous manual recalibration are avoided.
Pittsburgh-based PNC Financial Services Group, for example, developed a Customer Interaction Management system to deliver a centralized hub for managing all customer treatments across all channels. The AI-powered “brain” automatically analyzes customer data and the context of the inbound interaction, and then makes a decision on the Next-Best-Action based on this data.
This allows each recommended customer treatment — whether it is a cross-sell, purchase of a new product, or a needed service — to be delivered in real-time, automatically adjusting the process to match the situation.
The customer models used to drive the treatment strategies stay relevant with adaptive analytics that learn on the fly and adjust models automatically, relieving the bank from having to manually retool them whenever there are changes in customer behavior or market conditions.
Free Email Newsletter: Subscribe to The Financial Brand for Banking News & Trends

Using AI to Meet Customer Priorities
Brands are still telling customers about what they want to talk about, rather than what customers really want or need to know about. Research conducted by Pegasystems during the pandemic highlighted that approximately two-thirds of consumers don’t believe that brands care about their needs.
Centralized AI-powered decisionmaking can help address this issue and inject the necessary empathy back into customer interactions. By allowing organizations to choose from thousands of potential conversations based on live indications of customer need, it breaks down those barriers between sales and service to focus on making a more meaningful connection with a customer in the moment.
AI-powered decisionmaking can also help eliminate biases related to age, ethnicity or gender that can unintentionally creep into analytics and skew outcomes. Unchecked, these can result in regulatory violations, discriminatory customer engagements, and loss of public trust.
In highly regulated industries such as banking, centralized decisionmaking can be an invaluable tool to demonstrate why offers are being presented to specific customers and prove that no unconscious bias is at work. This is especially true when it comes to credit risk and ethical lending. Some solutions can now enable businesses to adapt their models and apply ethical AI decisionmaking to identify and avoid specific types of biases.