Andre Iervolino, director of digital marketing and research at Public Service Credit Union, is obsessed with Netflix.
While many focus on the unique subscription model Netflix has used to reinvent the entertainment industry, Iervolino is more interested in what’s happening behind the scenes — the engine that’s made Netflix successful.
With more than 81 million members in 190 countries, the internet television network has been a pioneer in digital channels. No two recommendations — even within the same household — are alike. It’s personalization on steroids.
The machine-learning algorithms used to decide what videos to recommend for each subscriber have been the driving force behind the Netflix experience. A combination of A/B testing focused on improving member retention coupled with offline experimentation using historical member engagement data has turned the Netflix recommendation engine into a work of art.
“Every interaction on the platform is logged into a database that is continuously learning about you through the content consumed to offer suggestions on the next best content,” he says. “Think about it. Algorithims are behind every single recommended video you see. Imagine how much value Netflix is able to gain from personalizing data gathered in terms of customer retention?”
This got Iervolino thinking about his credit union’s data. With $2 billion in assets and over 200,000 members, they had already amassed huge repositories of information. It was just sitting there, waiting to be tapped.
But like many financial institutions, you can get easily overwhelmed with the magnitude of it all. “How do you mine insights from trillions of bytes of data?” Iervolino says it doesn’t have to be that way. Here’s what he learned.
Read More: Winning in the Algorithmic Age – The ‘Big Bang’ of Bank Marketing
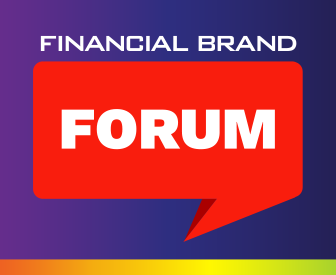
The Financial Brand Forum Kicks Off May 20th
Explore the big ideas, new innovations and latest trends reshaping banking at The Financial Brand Forum. Will you be there? Don't get left behind.
Read More about The Financial Brand Forum Kicks Off May 20th

The unfair advantage for financial brands.
Offering aggressive financial marketing strategies custom-built for leaders looking to redefine industry norms and establish market dominance.
1. Identify the business problems that you want to solve.
Examine everything that you think might benefit from machine learning — from product design and pricing, service strategies and marketing promotions. You’ll want to find the answers to who, what, why, when, where and how for each potential area you identify, then let that be the filters you use gather data and compile your business intelligence. That means asking the right questions, which will be unique for each financial institution. At PSCU, it started with asking who did the credit union want to target and why? That led to the next step.
2. The right metrics will drive data gathering.
Seeking the answers to those questions helped narrow the data search field allowing PSCU to draw on the most relevant information. Iervolino identified seven business problems/challenges the credit union needed to address:
- Increase the member lifetime value
- Increase member engagement
- Reduce member churn
- Increase profitability
- Increase loyalty index
- Increase Net Promoter Score
- Increase member participation
3. Identify and attribute which areas are impacted.
Tasked with addressing what PSCU should do next, a team of eight was assembled into they newly created “Digital Marketing, Research & Member Success Department.” Their task? To evaluate existing members — tenure of accounts, profitability, types of accounts held, etc. — then create a matrix that defined member lifetime value (MLV).
Members were then segmented into four conceptual categories with code names inspired by the Denver Broncos, the NFL home team for the Lone Tree, Colorado based credit union:
- “John Elway” — optimal MLV with checking, PFI status, high participation, mostly profitable long-term member.
- “Terrell Davis” — new member, with checking, high participation, PFI, mostly profitable, multiple services.
- “Peyton Manning” — at risk MLV, no checking or PFI status, long-term mostly unprofitable member.
- “Aaron Hernandez” — at risk MLV, low participation new member single/few services, no checking or PFI status mostly unprofitable.
“For us, our goal is to increase the member lifetime value and all that’s associated with it,” says Iervolino. “If we increase that, then we reduce member churn while increasing loyalty, member participation and that in turn positively impacts other metrics across our organization that helps improve the bottom line.”
With four different member personas identified, the team then designed a roadmap of outbound service calls across the members’ preferred channel offering the best cross-selling opportunities by life stage, deposit purchase intent, loan purchase intent, service satisfaction levels, referral likelihood, demographics, and other important psychographics elements.
“What is Netflix but a model that constantly cross-sells you?” Iervolino asks. “So we took a similar approach with our four member categories. The objective was to shift as many as possible into the ‘John Elway’ camp and maximize their member lifetime value.”
The data and intelligence PSCU was able to gather allowed for more personalized service calls and direct mailings.
“Measurement, tracking have been key,” says Iervolino. “Weekly team meetings are held to review and analyze what’s worked, improvement opportunities and the current goals.”
Iervolino likened it to an assembly line, as the department’s in-house research drives the digital marketing leads for optimal members success. Results then go back to research for analysis of audience size contacted, conversion rates, overall number of products adopted, services used, preferred channels, etc. Engagement metrics are compared month-over-month to determine member participation, loan and deposit growth, overall member profitability and develop and implement strategies to reduce member churn. The last step is to review the ROI in terms of the amount of hours spent on initiatives, average profit for campaigns and, ultimately, what the effort contributed to the credit union’s strategic goals.
Read More: The Use of AI in Banking is Set to Explode

4. Believe in the ROI.
Six months after launching what PSCU has dubbed their “Member Success Initiative”, the credit union had contacted a total of 7,903 members. Of those, 2,303 had increased engagement — either adopting or starting using a new product/service. That’s a conversion rate greater than 25%. Total loan production was $1.8 million, and new deposits topped $420,000. According to Iervolino, the ROI on the initiative was 13.16%.
PSCU also experimented with onboarding indirect members (those typically obtained through auto dealerships). Out of 6,434 indirect members contacted, 2,533 were successfully onboarded with additional products/service, a conversion rate of 39.4%. More than 1,700 indirect members adopted PSCU’s Home Banking service (roughly 30%). The credit union estimates that it is now converting 16% of indirect members to full-fledged retail status.
Being able to prove the ROI helps assuage any concerns in the C-suite about the investment and reallocation of resources.
“Maybe you start with a team of just two or three people,” Iervolino suggests. “If you can show the you are making money that positively impacts the bottom line, then it’s a matter of asking them to trust in the ROI that’s being created and that’s a risk worth taking.”
Iervolino says any financial institution can do this, regardless of their size.
“It’s about asking the right questions, and using the data you already have,” he explains. “You don’t have to make it overly complex with data governance and warehousing.”
5. Keep learning and scaling up.
“Our department’s focus on having existing and new members achieve optimal member lifetime value, and the results generated by identifying the right data to use — then acting on it — have been incredible,” Iervolino says.
Always looking ahead, Iervolino says the team will continue to reach out to members, building upon service calls by leveraging geofencing and retargeting initiatives.
Iervolino is also taking steps to make another dream a reality: creating a Match.com type algorithm that pairs members (and prospective members) with the best possible representative at the credit union. Factors that could drive the algorithm include the nature of the inquiry (sales or service), the type of product/service that someone is inquiring about, the preferred communications channel, and even their age and personality type.
To pull this off, Iervolino plans on tapping CodeChef, a not-for-profit educational initiative created by Directi, an Indian software company. CodeChef is a global programming community that fosters learning and friendly competition. Ierlovino likenes it to crowdsourcing for coding. He posts his problems on the website which goes out to CodeChef’s database of coders around the world who then compete to create a winning algorithm.
“Those solutions can then be used by our organization and it’s free,” he says. “It’s crowdsourcing at its best and a great option for those of us who may not have the resources to develop our own algorithms.”