The term “big data” stirs up plenty of excitement and discussion among financial marketers. Data (or information) — and our access to it — has exploded since the day Gutenberg rolled the first Bible off his printing press. Before that, all information was shared verbally, or was meticulously transcribed by hand. In the first 50 years after the printing press was introduced, 8 million books were printed. How significant was that? It was more books than all the scribes in Europe had created in the prior 1,000 years! But that’s nothing compared to the volumes of data we are currently creating.
In the year 2000, three-quarters of all data was analog. By 2013, analog comprised only 2% of all data; digital data exploded from a one-quarter share in 2000 to approximately 98% a mere 13 years later. That’s why today we talk about “digital overload.” And this is one of the main reasons so many businesses — including financial institutions — are as excited about big data opportunities as they are intimidated by the sheer scale of digital information that is now out there.
Where does all this digital data come from? It comes from you, your co-workers, friends, consumers… everyone. Like the engine exhaust trailing behind a running car, the average person generates a trail of digital data throughout the day. Use your cell phone. Visit a website. Follow an online search. Make purchases in-store or online. Every one of these actions creates data, data and more data. Consider these staggering examples:
The four biggest online storage services are estimated to warehouse a combined 1,200 petabytes. (That’s one million terabytes, and one terabyte is 1,000 gigabytes.)
- Every day, Facebook has 300 million new photos uploaded and 3 billion new comments posted.
- Over 6 hours of video is uploaded to YouTube every second.
- Twitter users generate 500 million new tweets per day.
- The Federal Reserve shows there are over 120 billion annual non-cash (i.e., trackable) payment transactions per year.
This plethora of information would overwhelm us if we tried to approach it with the same tools and methods used in the past. Traditionally, researchers used data to answer the question “why.” They would look for a causal effect in the data that would allow them to develop a model to predict future outcomes. But using big and non-traditional data makes it hard to apply causality when making decisions or predictions. Instead of addressing the “why,” big data addresses the “what.” In other words, a big data approach looks for outcomes and does not necessarily try to determine why those outcomes occur. (If you found out that the top 10% of your most profitable customers were all Bruce Springsteen fans, would you really care why? Or would you just start targeting more Bruce Springsteen fans?)
You probably heard about Google’s H1N1 flu study. In 2009, a new strain of flu, H1N1 (aka “swine flu”), appeared. The Centers for Decease Control and Prevention (CDC) traditionally relied on doctors to report any outbreaks. But their reports would typically trail several weeks behind the outbreak, which raised the question: was there a timelier way to detect — or even predict — outbreaks? Google engineers analyzed their more than 1.1 billion unique monthly searches and processed a staggering 450 million mathematical models testing search terms. Eventually they were able to successfully predict the spread of the H1N1 flu before there was any reported outbreak. In this instance, it was all about the “what.” What consumers searched for with more frequency told data engineers where outbreaks would happen next.
Unfortunately, after several years of accurate flu forecasts, the predictive model became less and less accurate. Why? There are multiple reasons this shift may have happened. It could have been that the news was full of other scary flu stories towards the end of 2012, which resulted in a lot of healthy people searching for flu information. Another explanation could be that even Google’s searches were biased. Would there be geographical markets where fewer people utilize Google, where some just talk to their pharmacist instead?
Along with big data comes a whole new set of risks, questions, and ethical issues. Because human beings both generate and study big data, the conclusions drawn won’t always be entirely accurate. There is room for error. Human error. Then there is “old” data — data that was collected under the presumption of privacy. And then there are issues about consumers’ level of comfort with how their information is being utilized. For instance, MasterCard has compiled data that shows when a driver fills his vehicle’s tank around 4:00 p.m., she or he is very likely to spend between $35 and $50 within the next hour at a supermarket or restaurant. Ethically speaking, how can the company use that information, and do so without triggering the “creepy factor” that trouble many consumers today?
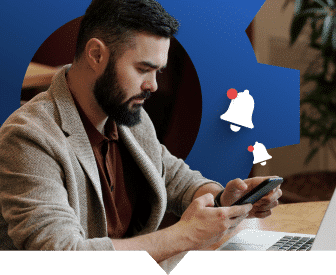
Instant Messaging. Instant Impact.
Connect with your customers and provide lightning-fast support as effortlessly as texting friends. Two-way SMS text messaging is no longer optional.
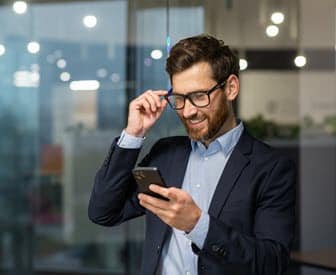
Navigating the Role of AI in Financial Institutions
83% of FI leaders agree investing in AI is essential for 2024 but how you leverage AI is instrumental in success and meeting customer expectations.
Read More about Navigating the Role of AI in Financial Institutions
Big Data in Banking
At the intersection of all these questions lurk some potentially major implications for the banking industry. Marketers are wondering how they can leverage these new data streams to grow new relationships and cultivate existing ones.
The truth is that marketers always need to be adding and complementing data sources to drive growth. In marketing, modeling has always been a key tool used to define and grow the core customer base. Just using your financial institution’s databases can arm you with some sizeable “big data” sets. When a certain segment of your customers love your brand, utilize your services, find you convenient and appreciate your value, the same will likely be true for their peers (aka “lookalikes”). Again, don’t trouble yourself with the “why” — as intellectually curious as you may be, it really doesn’t matter. All you need to care about is the “what” — what your ideal target audience(s) have in common.
New data sources are now available to enhance this. Companies like StreetLight have mobile- and GPS-based data that can be used to help financial institutions pick their next retail branch location. But mobile and GPS data also has other applications. We now live in a world where each consumer leaves a digital footprint through their GPS-enabled device. These devices can be utilized to determine traffic patterns for people who are more likely to utilize your services, online and in the branches. The fact is that these people are likely to be closer to you more often, so they are likelier to pick your organization when they are looking for a new financial product or solution.
We recently tested this notion with some surprising results. Integrating big data from 150 million mobile and GPS devices and hundreds of activity points from these sources allowed a better, more predictive targeting approach. Remember, though, conclusions drawn from big data are not infallible. As with all data, continued testing and verification is key.
Banking Transaction Data: The Holy Grail?
Your financial institution may or may not have tried to mine the accountholder data you maintain. But certainly there is a large amount of personal information available. Years ago, banking revolved around simple handwritten accounting ledgers. The collection of transaction data today has become far more sophisticated and efficient. Today’s systems are capable of reading the payee line on checks, endorsement information, and can interpret debit card transactions.
Your banking systems hold a wealth of highly-targeted leads for your sales and marketing teams, including:
- Commercial loans, including amounts borrowed, collateral properties and uses for the cash
- Merchant services, depicting who uses what services, how often, and in what amounts
- Brokerage/wealth management, with details about investments, savings balances, and beneficiaries
- Insurance, which like brokerage/wealth management services, offers personal information about the policyholder
Every retail or commercial product and service your financial institution offers dips into each individual’s personal or business lives. And this is extremely useful data. These days, if someone pays a mortgage held with another institution using your checking account, you should be able to know about it. If someone has an insurance product somewhere else, you should know about it. If they have a credit card with another institution, you should know. If they have a business loan somewhere else, you know it.

What Do You Do With the Data?
How about consultative sales? A customer could freely opt-in to your analytical service (driven by big data insights), then your staff could help them analyze that person’s financial behaviors and spending patterns. You could follow up with helpful recommendations and offers for other products and services.
There are also many ways financial marketers could use big data to turn their institutions’ websites into a 24-hour sales and engagement tool. Besides using data to refine targeting of banner ads on your website (promoting your own products and services), you could consider making advertising arrangements with local businesses. Look for purchases made at your business banking clients, and use that information to create various marketing partnerships (e.g., banner ads on your website, or something akin to a “merchant rewards program”).
Now, let’s make this a little more personal… Financial institutions know where their customers are spending their money. Within the bounds of ethics yet with an eye toward expanding your marketing reach, how can you leverage that data? How can you monetize this extremely valuable intel? Will financial institutions see data as the real value of offering checking accounts?
What about partnerships with organizations like Living Social or Groupon, who spend millions of dollars advertising products that may or may not apply to their subscribers? Would there be an option to provide a benefit to your customers that these organizations would be willing to pay for? Or, if it is not for a megabrand like Groupon, could it be something you explore with your own business customers?
Big data offers a lot of food for thought. You can be sure that other banks and credit unions are thinking about it right now.
Achim Griesel is Chief Operating Officer at Haberfeld Associates, a customer acquisition marketing and profitability consultant for community-based financial institutions in the country.