World War II movies often have a scene where entire villages turn out to cheer the victorious Allies as they rumble by in their jeeps, with people in the crowd waving American flags and women kissing the GIs. Everyone’s deliriously happy!
Stories about innovation in banking frequently have a similar vibe.
But banks and credit unions intending to step up their investment in innovation — especially artificial intelligence — have to be prepared for unhappy villagers.
Olga Tsubiks, director of strategic analytics and data science at Royal Bank of Canada, cited the steep learning curve for banking executives and employees after disruptive change arrives as one of the common points of contention.
“Innovation is hard,” Tsubiks said. “It’s walking uphill.”
More than that, the hardest part is not the technology. Tsubiks shared a story about the first AI project she led to illustrate why it’s the people who matter most.
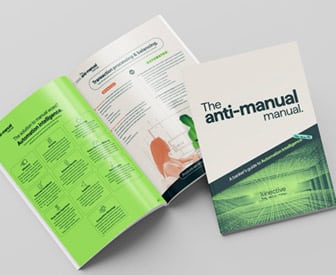
The New AI: A Banker’s Guide to Automation Intelligence
Manual tasks across channels is costly. And while AI is hot, there’s a simpler way to bring efficiency that many bankers have overlooked.
Read More about The New AI: A Banker’s Guide to Automation Intelligence

Why Industry Cloud for Banking?
PwC’s Industry Cloud for Banking helps deliver personalized products and services that today’s customers expect.
Rescuing a Failed AI Implementation
Being aware of the likely rough spots with a technology project can help ensure things don’t turn out to be more slog than celebration, Tsubiks said.
Speaking at Re-Work’s AI in Finance Summit in New York this spring, Tsubiks recalled a consulting assignment she handled before her time at RBC.
You might call it an AI rescue mission. It was her first experience leading a client assignment.
“Innovation is hard. It’s walking uphill.”
— Olga Tsubiks,
Royal Bank of Canada
The client firm had made a huge investment in new technology to completely revamp the operation. The traditional approaches used for years were slowing the organization down because they were too complex. Research determined systems driven by artificial intelligence were the answer. Or so it seemed…
A “proof of concept” was tested and the AI project got a green light to move forward. But implementation stalled.
Tsubiks was called in two years later, because “they couldn’t get it moving,” even after all that time. Meetings were happening, and deadlines were being set, but then extended.
Tsubiks said she initially thought some tweaks would be enough to get the AI implementation back on track for the bank. “But, of course, the problem wasn’t the AI solution,” she said. It was the people.
She ended up spending months talking to employees to figure that out, though.
The Reasons for Failure Offer the Insight Needed for Success
Out of the myriad of opinions she heard from employees, some key points emerged.
She found the three major challenges responsible for derailing the AI project were all people-related. These included:
1. Lack of buy-in
First, many people had no involvement in the proof of concept and didn’t understand what the point of it all was. Tsubiks said that they were so embedded in the old process that they couldn’t understand what their job would look like after the AI technology went live.
“They were used to the complexity of the old process and prided themselves on the fact that they could deal with it. So they didn’t really enjoy this new solution that extracted away the complexity and automated everything.”
— Olga Tsubiks, RBC
2. Daily demands
Second, the cadre of managers who had to get the work out resented the constant interruptions of the AI team trying to implement the new technology. Tsubiks said a common attitude was: “I don’t have time for your AI stuff. I have to keep the organization running. My old system is still there. So what’s the rush?”
3. Mixed messages from top executives
Finally, top management, though officially pushing for the changeover, gave mixed signals. Tsubiks said that they were very sensitive to errors or other untoward issues emerging from the AI’s attempted implementation.
“That drove some of the managers to be risk averse,” said Tsubiks, “and they would delay the migration of work to the new processes because they didn’t want to be caught by the risks.”
In the end, after nine months of fact-finding, Tsubiks was able to get things moving again. And the AI implementation became a success story.
Read more:
- Notably Quotable: What Banking and Tech Leaders Think of Generative AI
- What’s The Bank Tech To Invest In? And Which Might Never Pay Off?

Let’s Stop Fibbing About the Impact of Artificial Intelligence
Many of the issues that came up back then continue to be hurdles for AI implementations in banking, Tsubiks said.
The people impacted by that implementation were worried. “Their careers were evolving into something they’d never signed up to be,” she explained. “That is always very hard, but that AI impact on organizations is becoming more and more commonplace.”
Technology can make people feel not just uncomfortable, but threatened, as if they have lost control of their lives and livelihoods, Tsubiks said.
Fear is a rational — and common — reaction.
In this case it was also justified. “Sometimes entire departments were becoming obsolete. So they needed to be transformed so they could engage in different ways,” she said. “But sometimes there was just no space for them anymore, unfortunately.”
“When people’s salaries are very much tied to doing things in certain ways, it’s very hard to get them to think about something new and to try something new.”
— Olga Tsubiks, RBC
AI engineers and data scientists typically aren’t good at handling the human element of implementations, Tsubiks said. Even she favors getting into the nitty-gritty of innovation and addressing a need, rather than stepping back to take in the bigger picture.
Genuine empathy and understanding are essential when interacting with affected employees, Tsubiks stressed. Generally AI implementation in banking means major changes somewhere in the organization and there is a necessary balance between the present and the future.
“R&D is so important because it is focusing on what the organization is going to be tomorrow,” said Tsubiks.
In the research and development department, “they’re not worried about running the day-to-day business and taking care of customers today,” she said. “Investments in R&D are almost like insurance for making sure the company stays operational” in the future.
Tsubiks added that in her experience, leaders at all levels who can make decisions quickly and be authentic about why they’ve made those decisions tend to be those who facilitate timely innovation.
Read more:
- 3 Strategies for Enterprise AI Success That Are Tried and ‘Truist’
- Digging Into KeyBank’s Conversational AI Strategy
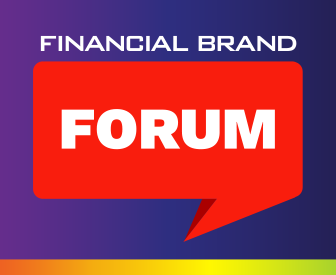
The Financial Brand Forum Kicks Off May 20th
Explore the big ideas, new innovations and latest trends reshaping banking at The Financial Brand Forum. Will you be there? Don't get left behind.
Read More about The Financial Brand Forum Kicks Off May 20th
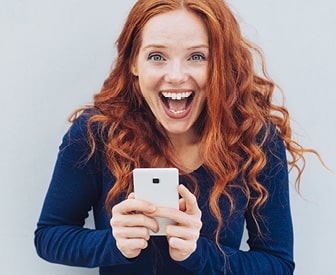
Redefining CX Through Innovative Social Media Strategies for Financial Services
Learn how Sprinklr is redefining success in the financial sector by harnessing the potential of tailored content and personalized engagement.
Read More about Redefining CX Through Innovative Social Media Strategies for Financial Services
3 Keys to Successful AI Implementation in Banks
Getting new approaches off the ground begins with the ideas themselves, which, Tsubiks said, can come from anywhere in a banking institution.
Then feedback from an institution’s innovation center or fintech lab can refine the ideas.
“It’s almost like a roadmap,” said Tsubiks. “You begin with the idea and what it’s going to take to create a prototype or a proof of concept. And then, what could it become one day? It could become a product, it could become a service, it could become a change to your process, or it could become a different line of business within the organization — or maybe even a different company.”
Empowering employees across the organization to provide input can help. Tsubiks said that beats the alternative of having AI experts trolling around the bank offering their expertise, like itinerant contractors.
Want to go deep on AI best practices for banks?
Attend our AI Masterclass — Unlocking the Power of Artificial Intelligence in Banking — at The Financial Brand Forum 2024 on May 20-22 in Las Vegas. Led by Ron Shevlin, chief research officer at Cornerstone, this three-hour workshop will be jam-packed with lessons learned from industry leaders and real-world case studies.
For more information and to register, check out the Forum website.
Tsubiks has three main pieces of advice for successfully implementing artificial intelligence projects:
1. Think about the “real world” use of an idea, not “use cases.”
She said it’s more important to weigh the value that the idea can create rather than simply move ahead with building a demo.
2. Stop thinking about how artificial intelligence fits into your bank’s business strategy.
Today’s strategy exists for today’s bank, said Tsubiks. Innovation thinking, if framed for banking as it is now, will likely result in incremental changes, perhaps with an occasional big idea from outside banking.
In other words, fitting innovation thinking to the present strategy will not produce major innovation, she insisted.
3. Recognize — and deal with — challenges such as security or compliance issues up front.
Don’t put that off. Waiting until later to worry about the details of an AI implementation only creates roadblocks at the crucial point when things should be humming along.
“Think about these things before you actually get your project working,” advised Tsubiks. “If you don’t envision what the problems are going to be, it will take you years to get through them and get to adoption.”
The danger is: “By the time you’re done implementing your innovation, it will be too little, too late.”
See more coverage of artificial intelligence in banking.